Expertise
In the following is given some examples from the 8 different topics mentioned. Unfortunately, most of the work has been confidential, but the chosen examples should give an idea of the interdisciplinary nature of biosimulation.
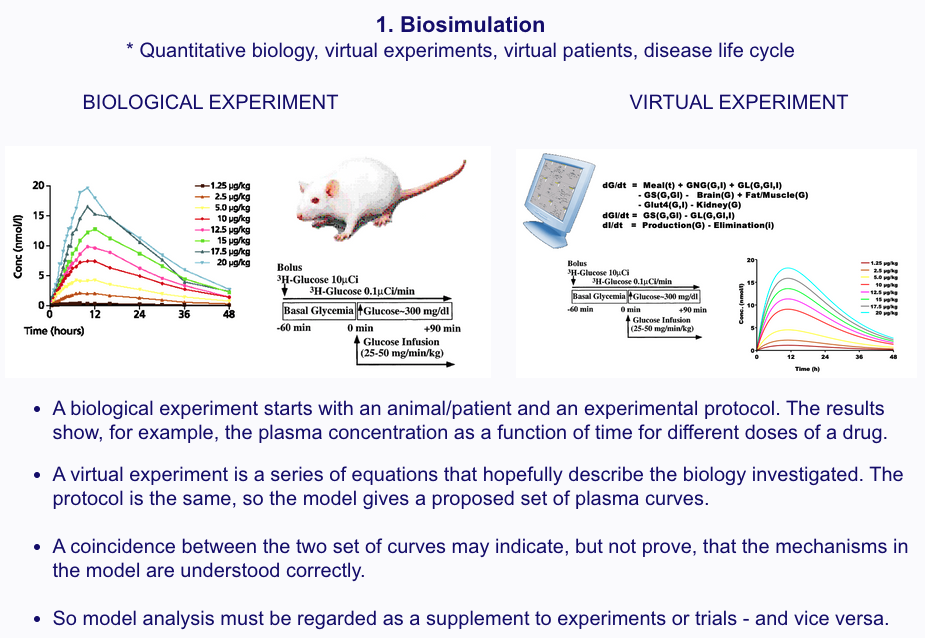
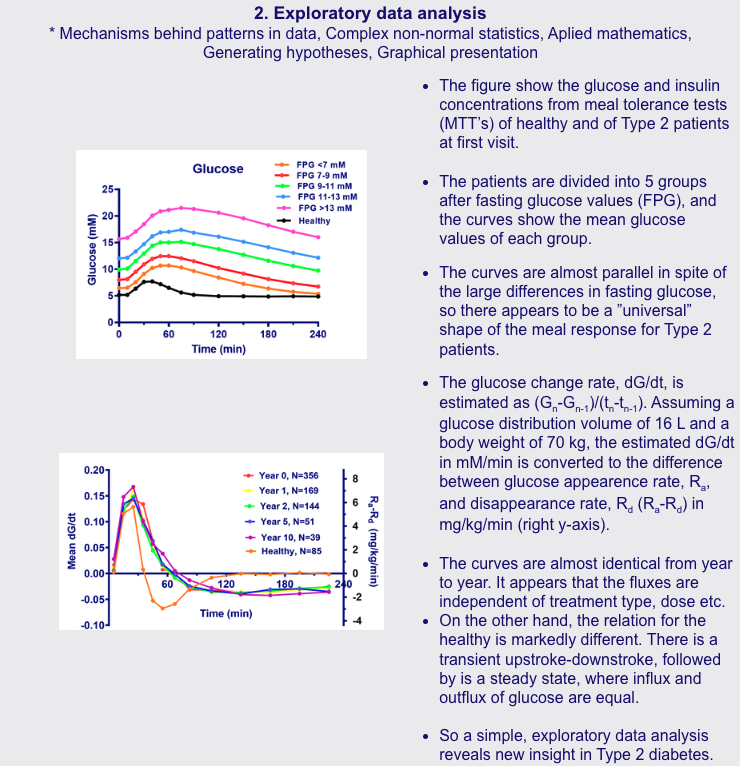
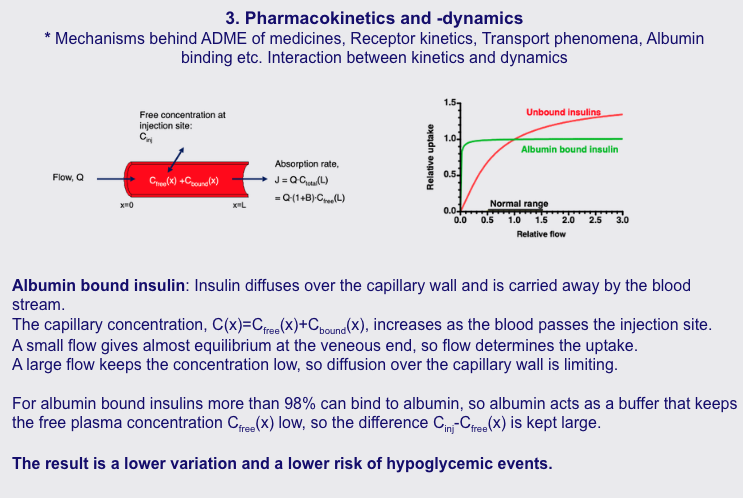
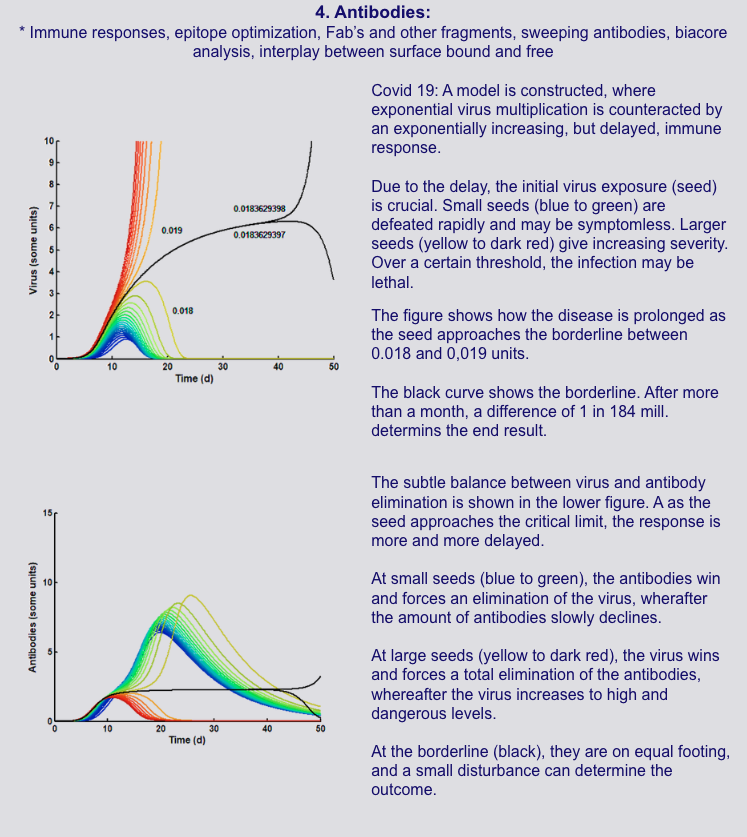

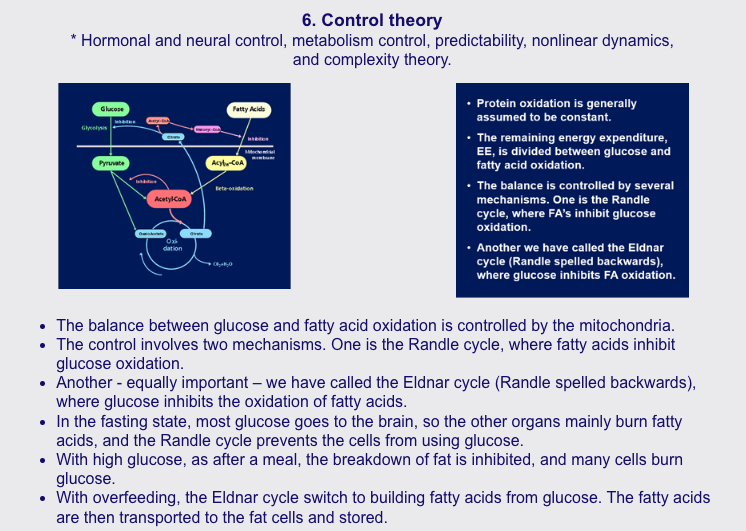


Expertise
In the following is given some examples from the 8 different topics mentioned. Unfortunately, most of the work has been confidential, but the chosen examples should give an idea of the interdisciplinary nature of biosimulation.